NerfAcc Documentation¶
NerfAcc is a PyTorch Nerf acceleration toolbox for both training and inference. It focus on efficient sampling in the volumetric rendering pipeline of radiance fields, which is universal and plug-and-play for most of the NeRFs. With minimal modifications to the existing codebases, Nerfacc provides significant speedups in training various recent NeRF papers. And it is pure Python interface with flexible APIs!
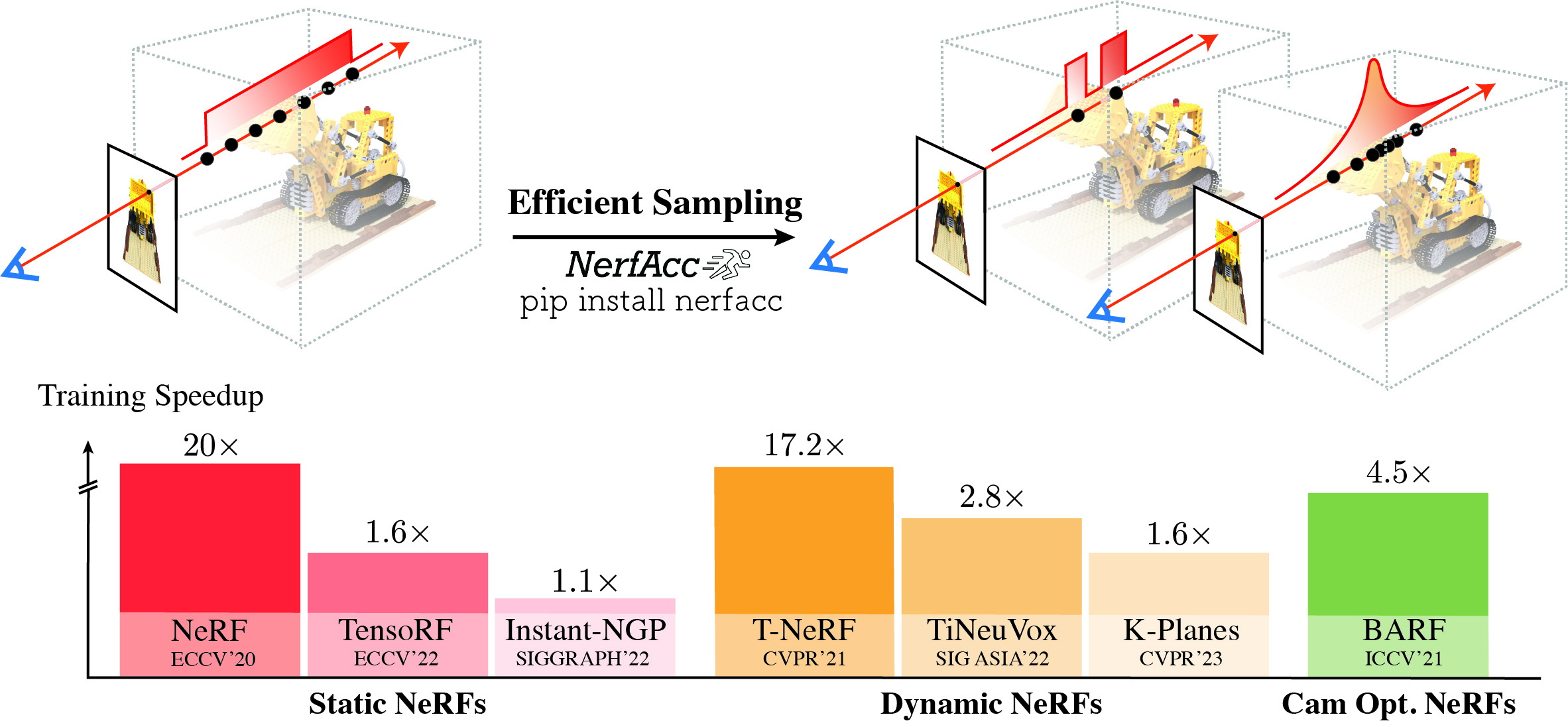
Note
Though this repo only contains examples for single scene optimization,
we believe generalizable NeRFs across multiple scenes can also be accelerate with our
nerfacc.PropNetEstimator
. Examples will be added soon.
Installation:¶
Dependence: Please install Pytorch first.
The easist way is to install from PyPI. In this way it will build the CUDA code on the first run (JIT).
$ pip install nerfacc
Or install from source. In this way it will build the CUDA code during installation.
$ pip install git+https://github.com/nerfstudio-project/nerfacc.git
We also provide pre-built wheels covering major combinations of Pytorch + CUDA versions. See our Github README for what we support.
// e.g. torch 1.13.0 + CUDA 11.7
$ pip install nerfacc -f https://nerfacc-bucket.s3.us-west-2.amazonaws.com/whl/torch-1.13.0_cu117.html
Usage:¶
The idea of NerfAcc is to perform efficient volumetric sampling with a computationally cheap estimator to discover surfaces. So NerfAcc can work with any user-defined radiance field. To plug the NerfAcc rendering pipeline into your code and enjoy the acceleration, you only need to define two functions with your radience field.
sigma_fn: Compute density at each sample. It will be used by the estimator (e.g.,
nerfacc.OccGridEstimator
,nerfacc.PropNetEstimator
) to discover surfaces.rgb_sigma_fn: Compute color and density at each sample. It will be used by
nerfacc.rendering()
to conduct differentiable volumetric rendering. This function will receive gradients to update your radiance field.
An simple example is like this:
import torch
from torch import Tensor
import nerfacc
radiance_field = ... # network: a NeRF model
rays_o: Tensor = ... # ray origins. (n_rays, 3)
rays_d: Tensor = ... # ray normalized directions. (n_rays, 3)
optimizer = ... # optimizer
estimator = nerfacc.OccGridEstimator(...)
def sigma_fn(
t_starts: Tensor, t_ends:Tensor, ray_indices: Tensor
) -> Tensor:
""" Define how to query density for the estimator."""
t_origins = rays_o[ray_indices] # (n_samples, 3)
t_dirs = rays_d[ray_indices] # (n_samples, 3)
positions = t_origins + t_dirs * (t_starts + t_ends)[:, None] / 2.0
sigmas = radiance_field.query_density(positions)
return sigmas # (n_samples,)
def rgb_sigma_fn(
t_starts: Tensor, t_ends: Tensor, ray_indices: Tensor
) -> Tuple[Tensor, Tensor]:
""" Query rgb and density values from a user-defined radiance field. """
t_origins = rays_o[ray_indices] # (n_samples, 3)
t_dirs = rays_d[ray_indices] # (n_samples, 3)
positions = t_origins + t_dirs * (t_starts + t_ends)[:, None] / 2.0
rgbs, sigmas = radiance_field(positions, condition=t_dirs)
return rgbs, sigmas # (n_samples, 3), (n_samples,)
# Efficient Raymarching:
# ray_indices: (n_samples,). t_starts: (n_samples,). t_ends: (n_samples,).
ray_indices, t_starts, t_ends = estimator.sampling(
rays_o, rays_d, sigma_fn=sigma_fn, near_plane=0.2, far_plane=1.0,
early_stop_eps=1e-4, alpha_thre=1e-2,
)
# Differentiable Volumetric Rendering.
# colors: (n_rays, 3). opaicity: (n_rays, 1). depth: (n_rays, 1).
color, opacity, depth, extras = nerfacc.rendering(
t_starts, t_ends, ray_indices, n_rays=rays_o.shape[0], rgb_sigma_fn=rgb_sigma_fn
)
# Optimize: Both the network and rays will receive gradients
optimizer.zero_grad()
loss = F.mse_loss(color, color_gt)
loss.backward()
optimizer.step()
Links:¶
Methodology
Python API
Example Usages and Benchmarks
Projects